AI Analytics
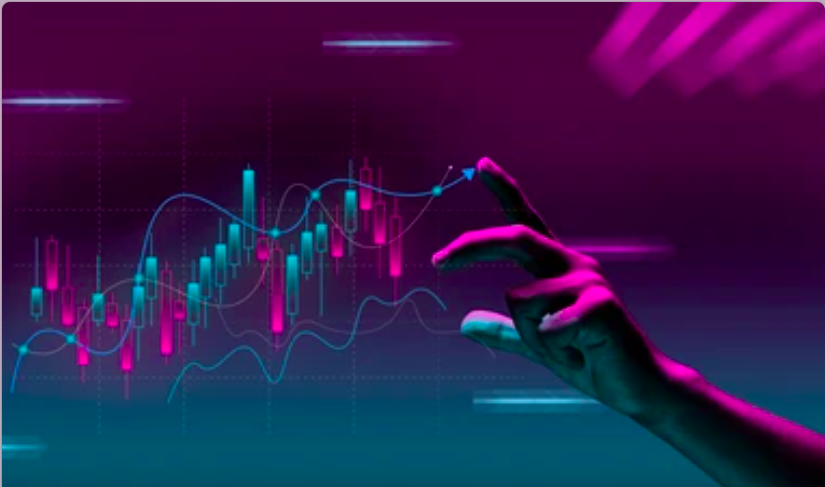
What is AI analytics
AI analytics or AI-driven analytics, also known as artificial intelligence-driven analytics, refers to the utilization of artificial intelligence (AI) technologies and techniques to analyze data and extract valuable insights automatically. This advanced approach combines the power of AI algorithms with traditional analytics methods to enhance the accuracy, speed, and depth of data analysis.
In AI-driven analytics, machine learning algorithms are employed to process vast amounts of data, identify patterns, trends, and anomalies, and generate predictive models without the need for human intervention. These algorithms can adapt and learn from data over time, continuously improving their predictive capabilities and uncovering new insights that may not be apparent through manual analysis alone.
Why is AI analytics important?
- Enhanced Decision-Making: By leveraging AI algorithms, organizations can analyze vast datasets quickly and accurately, empowering decision-makers to make informed choices based on data-driven insights rather than relying solely on intuition or past experiences. This leads to more effective and efficient decision-making across various business functions.
- Predictive Capabilities: AI-driven analytics enables predictive modeling by identifying patterns, trends, and correlations within data. This predictive capability allows businesses to anticipate future events, trends, and customer behaviors, enabling proactive strategies and risk mitigation measures.
- Improved Efficiency: With AI-driven analytics automating data processing, analysis, and reporting tasks, organizations can significantly improve operational efficiency and productivity. By reducing manual effort and human error, AI-driven analytics streamlines workflows, saves time, and frees up resources for more strategic activities.
- Competitive Advantage: Organizations that embrace AI-driven analytics gain a competitive edge by leveraging data insights to innovate, optimize processes, and deliver superior products or services. By staying ahead of market trends and customer preferences, businesses can adapt quickly to changing conditions and outperform competitors.
- Personalized Customer Experiences: AI-driven analytics enables businesses to better understand their customers' preferences, behaviors, and needs. By analyzing customer data, organizations can personalize marketing campaigns, product recommendations, and customer interactions, leading to higher customer satisfaction and loyalty.
- Risk Management: AI-driven analytics plays a critical role in identifying and mitigating risks across various business domains, including fraud detection, cybersecurity, and compliance. By analyzing historical data and detecting anomalies or irregularities, organizations can proactively address potential risks and safeguard their assets and reputation.
- Innovation and Growth: AI-driven analytics fosters innovation by uncovering new insights, opportunities, and market trends that may not be apparent through traditional analysis methods. By harnessing the power of AI to explore data from diverse sources, organizations can identify untapped markets, develop innovative products or services, and drive sustainable growth.
Use Cases of AI-Analytics
- Predictive Maintenance: AI-driven analytics is utilized in manufacturing industries to predict equipment failures and schedule maintenance proactively. By analyzing historical data and real-time sensor readings, AI algorithms can identify patterns indicative of potential equipment breakdowns, enabling businesses to conduct timely maintenance and prevent costly downtime.
- Customer Segmentation and Personalization: In e-commerce and retail, AI-driven analytics is employed to segment customers based on their preferences, behaviors, and purchase history. By analyzing vast amounts of customer data, businesses can create personalized marketing campaigns, product recommendations, and tailored shopping experiences, leading to higher customer satisfaction and increased sales.
- Fraud Detection and Prevention: Financial institutions leverage AI-driven analytics to detect and prevent fraudulent activities such as credit card fraud, identity theft, and money laundering. By analyzing transaction patterns, user behavior, and other relevant data points, AI algorithms can identify suspicious activities in real-time and alert authorities or block transactions to mitigate financial losses.
- Healthcare Diagnostics: In healthcare, AI-driven analytics aids in medical diagnostics by analyzing patient data, including medical records, imaging scans, and genetic information. AI algorithms can assist healthcare professionals in diagnosing diseases, predicting patient outcomes, and recommending personalized treatment plans based on individual patient profiles, leading to more accurate diagnoses and improved patient care.
- Demand Forecasting: Retail and supply chain industries utilize AI-driven analytics for demand forecasting to optimize inventory management and supply chain operations. By analyzing historical sales data, market trends, and external factors such as weather and holidays, AI algorithms can predict future demand with greater accuracy, enabling businesses to stock the right products in the right quantities and minimize stockouts or overstock situations.
- Churn Prediction in SaaS: In the SaaS industry, AI-driven analytics is applied to predict customer churn and identify at-risk customers who are likely to cancel their subscriptions. By analyzing user engagement metrics, product usage patterns, and customer feedback, AI algorithms can identify early warning signs of churn and enable SaaS companies to take proactive measures, such as targeted marketing campaigns or product enhancements, to retain customers and reduce churn rates.
AI analytics Pros
- Enhanced Insights: AI-driven analytics harnesses advanced algorithms to process vast amounts of data quickly and accurately, uncovering valuable insights that may not be apparent through traditional analysis methods.
- Predictive Capabilities: AI algorithms have the ability to predict future trends, behaviors, and outcomes based on historical data patterns, enabling businesses to make proactive decisions and mitigate potential risks.
- Automation: AI-driven analytics automates repetitive tasks such as data processing, pattern recognition, and report generation, freeing up human resources to focus on more strategic and creative aspects of business operations.
- Scalability: AI-driven analytics solutions are highly scalable, capable of handling large volumes of data and adapting to changing business needs without significant manual intervention or infrastructure upgrades.
- Personalization: AI-driven analytics enables businesses to deliver personalized experiences to customers by analyzing individual preferences, behaviors, and interactions, leading to higher customer satisfaction and loyalty.
AI analytics Cons
- Data Privacy and Security Concerns: AI-driven analytics relies heavily on data, raising concerns about data privacy, security, and ethical use. Mishandling of sensitive information or data breaches could result in legal and reputational damage for businesses.
- Complexity and Technical Expertise: Implementing and managing AI-driven analytics solutions requires specialized technical skills and expertise, which may be challenging for organizations lacking in-house talent or resources.
- Bias and Fairness Issues: AI algorithms may exhibit biases inherent in the data they are trained on, leading to unfair or discriminatory outcomes, particularly in sensitive areas such as hiring, lending, and criminal justice.
- Interpretability and Transparency: AI models often operate as "black boxes," making it difficult to interpret how they arrive at their decisions. Lack of transparency can undermine trust and confidence in AI-driven analytics systems, especially in high-stakes scenarios.
- Cost and ROI: While AI-driven analytics has the potential to deliver significant value to businesses, the initial investment in technology, infrastructure, and talent acquisition can be substantial. Achieving a positive return on investment (ROI) may take time and require careful planning and execution.
Differences between Traditional and AI Analytics
- Process: Traditional analytics often rely on manual data processing, analysis, and reporting methods, whereas AI analytics leverage automated algorithms and machine learning techniques to process vast amounts of data quickly and accurately.
- Insights Generation: Traditional analytics typically generate insights based on predefined rules and queries, while AI analytics can uncover complex patterns, trends, and correlations in data that may not be apparent to human analysts.
- Scalability: Traditional analytics may face limitations in scalability due to manual processes and resource constraints, whereas AI analytics solutions are highly scalable and capable of handling large volumes of data with minimal human intervention.
- Decision-Making: Traditional analytics provide historical or descriptive insights that require human interpretation and decision-making, whereas AI analytics offer predictive and prescriptive insights that can inform real-time decision-making and automate certain actions.
- Adaptability: Traditional analytics methods may struggle to adapt to changing data sources or business environments, whereas AI analytics can continuously learn and evolve based on new data inputs, improving accuracy and relevance over time.
- Data Complexity: Traditional analytics may struggle to process and derive insights from highly complex and unstructured data sources, such as text, images, and videos, whereas AI analytics excel in handling diverse data formats and extracting meaningful insights through techniques like natural language processing and computer vision.
- Resource Efficiency: Traditional analytics often require significant human resources and time to collect, clean, and analyze data manually, leading to higher costs and longer processing times, whereas AI analytics automate many of these tasks, reducing the need for manual intervention and improving resource efficiency.
In conclusion, EnqDB stands at the forefront of leveraging AI-driven analytics to revolutionize data analysis and decision-making processes. By harnessing the power of artificial intelligence, EnqDB offers innovative solutions that enable organizations to extract actionable insights from their data quickly and efficiently.
With EnqDB as a trusted partner, organizations can unlock the full potential of their data assets, driving growth, efficiency, and competitive advantage in today's data-driven world.