Data Analytics
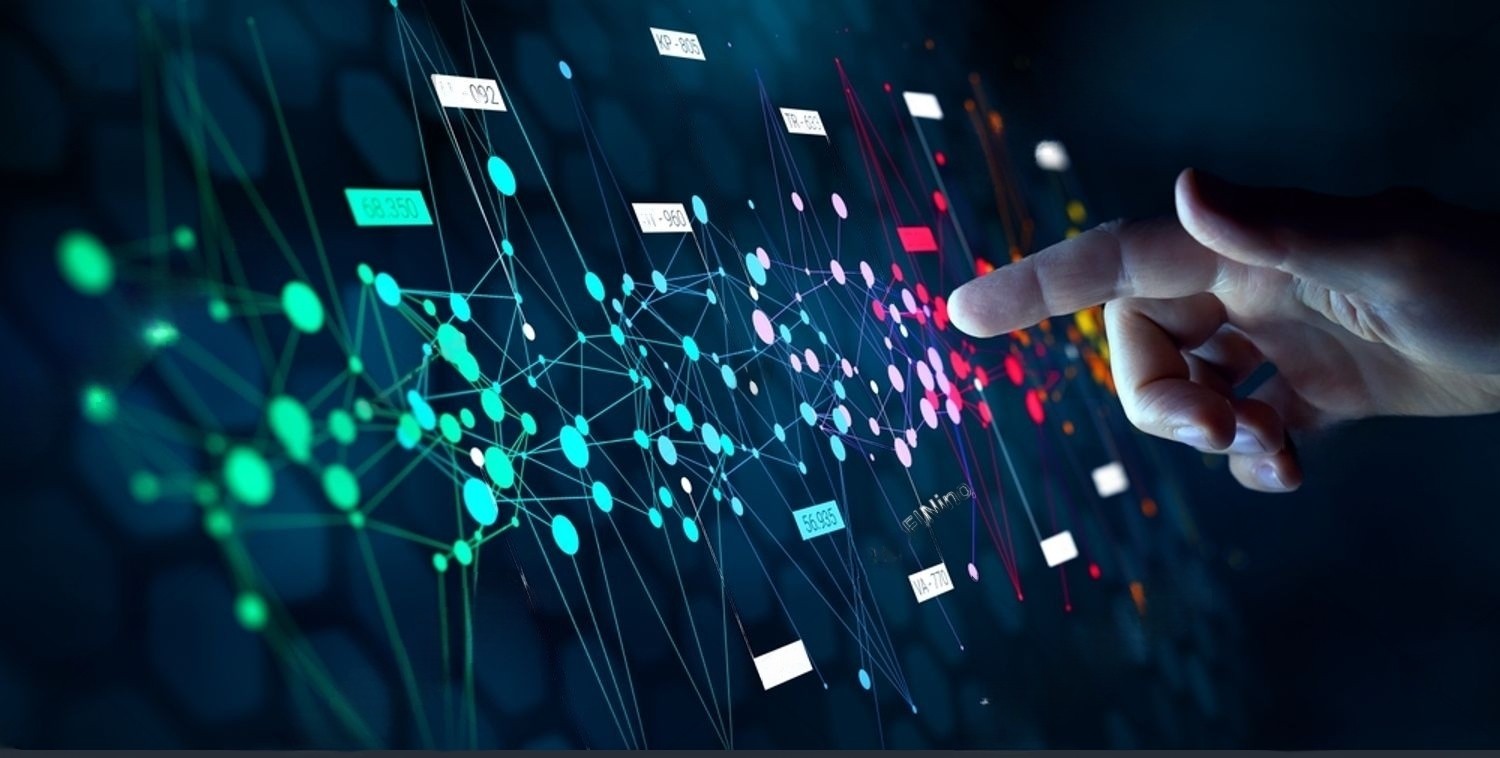
What is Data analytics
Data analytics is the science of analyzing raw data to extract meaningful information. It involves a range of techniques from statistical analysis and machine learning to data mining and predictive modeling. The goal is to uncover patterns, draw conclusions, and ultimately drive strategic decisions that can improve efficiency, increase profitability, and enhance customer satisfaction.
Importance of Data Analytics
Data analytics is an essential tool for businesses in the modern world. It enables organizations to make data-driven decisions, optimize operations, and gain a competitive advantage. Here are some key reasons why data analytics is important:
- Informed Decision-Making: Data analytics provides valuable insights that help businesses make informed decisions. By analyzing data from various sources, companies can identify trends, uncover patterns, and understand customer behavior, leading to more strategic and effective decision-making.
- Improved Efficiency: Through data analytics, businesses can streamline their operations and improve efficiency. By analyzing operational data, companies can identify bottlenecks, optimize processes, and reduce costs, resulting in increased productivity and profitability.
- Enhanced Customer Insights: Data analytics allows businesses to gain a deeper understanding of their customers. By analyzing customer data, companies can identify preferences, behaviors, and needs, enabling them to tailor products, services, and marketing strategies to better meet customer demands and enhance satisfaction.
- Risk Management: Data analytics plays a crucial role in risk management by helping businesses identify potential risks and take proactive measures to mitigate them. By analyzing historical data and identifying patterns, companies can predict and manage risks more effectively, reducing financial losses and ensuring business continuity.
- Innovation and Growth: Data analytics fosters innovation by providing insights that drive new ideas and opportunities. By analyzing market trends and customer feedback, businesses can develop innovative products and services, explore new markets, and achieve sustainable growth.
- Competitive Advantage: In today's competitive business landscape, data analytics provides a significant competitive advantage. Companies that leverage data analytics to gain insights and make data-driven decisions are better positioned to outperform their competitors, adapt to changing market conditions, and seize new opportunities.
Key Techniques in Data Analytics
Data analytics encompasses a range of techniques that help organizations extract valuable insights from data. Here are some of the key techniques used in data analytics:
- Descriptive Analytics: Descriptive analytics involves summarizing and interpreting historical data to understand what has happened in the past. Techniques such as data aggregation, data mining, and data visualization are used to present data in a meaningful way, providing insights into trends, patterns, and anomalies.
- Diagnostic Analytics: Diagnostic analytics focuses on understanding why something happened. By using techniques such as drill-down, data discovery, and correlation analysis, businesses can identify the root causes of issues and uncover hidden relationships between variables, leading to deeper insights.
- Predictive Analytics: Predictive analytics uses statistical models and machine learning algorithms to predict future outcomes based on historical data. Techniques such as regression analysis, time series analysis, and classification help organizations forecast trends, anticipate customer behavior, and make informed decisions about future actions.
- Prescriptive Analytics: Prescriptive analytics goes beyond predicting future outcomes to recommend actions that can influence those outcomes. Techniques such as optimization, simulation, and decision analysis provide actionable recommendations that help businesses achieve their objectives and improve performance.
- Exploratory Data Analysis (EDA): EDA involves using visual and statistical techniques to explore data and uncover patterns, relationships, and anomalies. By using techniques such as scatter plots, histograms, and box plots, analysts can gain a better understanding of the data and generate hypotheses for further analysis.
- Text Analytics: Text analytics involves analyzing unstructured text data to extract meaningful insights. Techniques such as natural language processing (NLP), sentiment analysis, and keyword extraction help businesses understand customer feedback, monitor brand sentiment, and gain insights from large volumes of text data.
- Data Visualization: Data visualization involves presenting data in graphical or visual formats to make complex data more accessible and understandable. Techniques such as charts, graphs, and dashboards enable businesses to quickly grasp insights, identify trends, and communicate findings effectively.
What is Big Data Analytics?
Big data analytics refers to the process of examining large and varied data sets to uncover hidden patterns, correlations, market trends, customer preferences, and other useful information. This comprehensive analysis helps organizations make informed business decisions. Here are the key aspects of big data analytics:
- Volume: Big data analytics deals with massive amounts of data generated from various sources, such as social media, sensors, transactions, and more. This volume of data requires advanced storage, processing, and analytical technologies to handle and analyze efficiently.
- Variety: The data analyzed in big data analytics comes in different formats, including structured data (like databases), semi-structured data (like XML files), and unstructured data (like text, images, and videos). Handling this variety of data requires versatile analytical tools and techniques.
- Velocity: Big data is generated at high speeds, often in real-time. The ability to process and analyze data rapidly as it is created enables organizations to make timely decisions and gain real-time insights into their operations and markets.
- Veracity: The accuracy and reliability of big data are crucial for meaningful analysis. Data veracity involves ensuring data quality by cleaning and validating data, which helps in reducing noise and enhancing the reliability of the analysis results.
- Value: The ultimate goal of big data analytics is to extract valuable insights that can drive strategic business decisions. By analyzing large data sets, organizations can uncover patterns and trends that provide a competitive advantage and inform business strategy.
- Advanced Analytics: Big data analytics employs advanced analytical techniques such as machine learning, data mining, predictive modeling, and artificial intelligence. These techniques help in uncovering complex patterns and making accurate predictions from large and diverse data sets.
- Scalability: The infrastructure for big data analytics must be scalable to accommodate the growing volume, variety, and velocity of data. Cloud computing and distributed computing frameworks like Hadoop and Spark enable scalable storage and processing of big data.
- Real-Time Processing: Real-time big data analytics allows organizations to process and analyze data as it is generated. This capability is critical for applications that require immediate insights, such as fraud detection, stock trading, and real-time customer engagement.
Career in Data Analytics
A career in data analytics offers a wide range of opportunities across various industries, driven by the growing importance of data-driven decision-making. Here are some key aspects of pursuing a career in data analytics:
- High Demand: With the increasing volume of data generated daily, there is a high demand for skilled data analysts who can interpret and leverage this data to provide actionable insights. Companies across sectors such as finance, healthcare, retail, and technology are actively seeking data analytics professionals.
- Versatile Skill Set: A career in data analytics requires a diverse set of skills, including statistical analysis, data visualization, programming, and domain-specific knowledge. Proficiency in tools and languages such as Python, R, SQL, and Tableau is highly valuable in this field.
- Career Progression: Data analytics offers clear career progression paths. Starting as a data analyst, professionals can advance to roles such as data scientist, data engineer, and business intelligence analyst. With experience and expertise, they can move into senior positions like data analytics manager or chief data officer.
- Impactful Work: Data analytics professionals play a crucial role in driving strategic decisions and business outcomes. By uncovering insights from data, they help organizations optimize operations, improve customer experiences, and identify new opportunities, making their work highly impactful.
- Continuous Learning: The field of data analytics is constantly evolving, with new tools, techniques, and technologies emerging regularly. A career in data analytics involves continuous learning and staying updated with industry trends, which can be intellectually stimulating and rewarding.
- Interdisciplinary Nature: Data analytics is inherently interdisciplinary, requiring collaboration with professionals from various domains such as business, IT, and operations. This interdisciplinary nature allows for a broader understanding of business problems and innovative solutions.
Conclusion
In conclusion, EnqDB stands at the forefront of leveraging Data analytics to revolutionize decision-making processes. By harnessing the power of artificial intelligence, EnqDB offers innovative solutions that enable organizations to extract actionable insights from their data quickly and efficiently.
With EnqDB as a trusted partner, organizations can unlock the full potential of their data assets, driving growth, efficiency, and competitive advantage in today's data-driven world.