Data Discovery
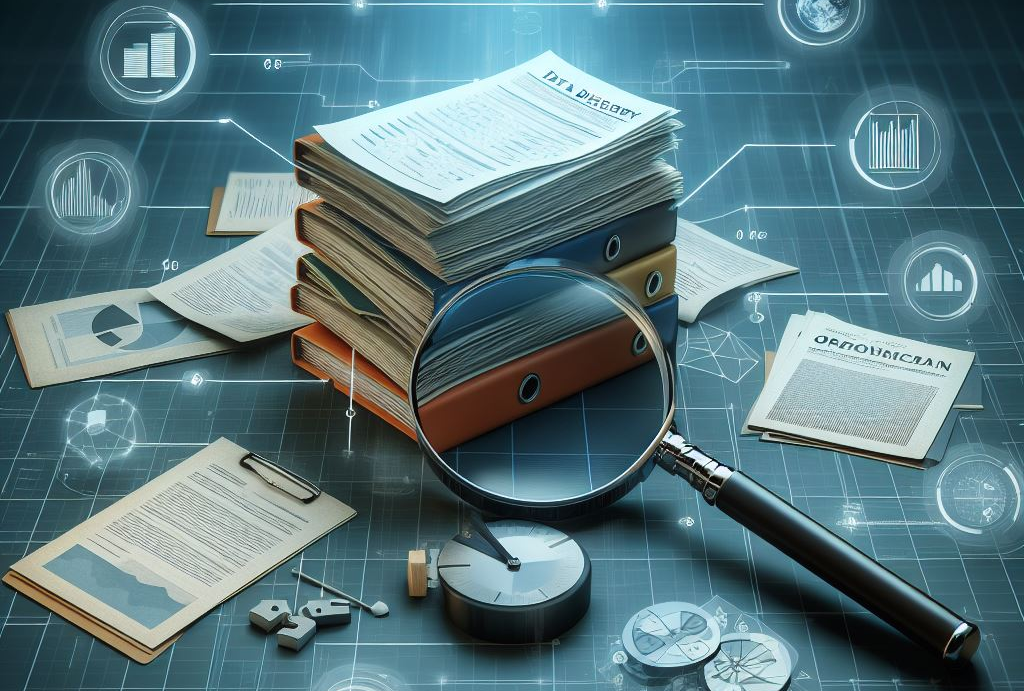
What is Data Discovery?
Data discovery in business intelligence (BI) involves the systematic exploration of datasets or data sources to identify patterns, trends, and insights. It encompasses the utilization of various tools and methodologies to delve into data and extract valuable information that aids decision-making and shapes business strategy.
Through data discovery, users can dynamically interact with data, conducting analyses to address specific inquiries or unearth novel opportunities. This process empowers users to engage with data interactively, facilitating the identification of pertinent insights crucial for informed decision-making and strategic planning.
Importance of Data Discovery
- Informing Strategic Decision-Making: Data discovery empowers organizations to make strategic decisions based on factual insights rather than intuition or guesswork. By uncovering trends, patterns, and correlations within data sets, decision-makers can gain a comprehensive understanding of market dynamics, customer behavior, and competitive landscapes. This enables them to formulate informed strategies, allocate resources effectively, and seize opportunities for growth and expansion.
- Driving Innovation and Agility: In today's rapidly evolving business environment, innovation and agility are critical for staying ahead of the curve. Data discovery fuels innovation by enabling organizations to identify emerging trends, customer preferences, and market opportunities. By leveraging data-driven insights, businesses can innovate new products, services, and business models that meet evolving customer needs and preferences, driving competitive differentiation and sustainable growth.
- Enhancing Customer Engagement: Data discovery plays a vital role in enhancing customer engagement and satisfaction by enabling organizations to gain deeper insights into customer preferences, behaviors, and sentiments. By analyzing customer data, businesses can personalize interactions, tailor offerings to individual needs, and deliver seamless omnichannel experiences. This fosters stronger customer relationships, increases brand loyalty, and drives revenue growth in the long term.
- Optimizing Operational Efficiency: Data discovery helps organizations optimize operational efficiency by identifying inefficiencies, bottlenecks, and areas for improvement across business processes. By analyzing operational data, businesses can streamline workflows, automate repetitive tasks, and optimize resource allocation to maximize productivity and minimize costs. This enables organizations to operate more efficiently, achieve higher levels of performance, and drive bottom-line results.
- Enabling Data-Driven Culture: Data discovery fosters a data-driven culture within organizations, where data is valued as a strategic asset and used to drive decision-making at all levels. By democratizing data access and empowering employees with self-service analytics tools, organizations can cultivate a culture of data literacy, curiosity, and accountability. This encourages data-driven decision-making, fosters collaboration across departments, and drives continuous improvement and innovation.
Data Discovery Categories
- Exploratory Data Analysis: This involves the initial exploration and visualization of data to gain a preliminary understanding of its characteristics, distributions, and relationships.
- Pattern Recognition: Data discovery tools can identify recurring patterns, anomalies, and trends within large datasets, helping users uncover valuable insights and make data-driven decisions.
- Predictive Modeling: Leveraging statistical algorithms and machine learning techniques, data discovery tools can forecast future trends, outcomes, or behaviors based on historical data patterns.
- Root Cause Analysis: By drilling down into data sets, users can trace the underlying causes of specific outcomes or events, enabling organizations to address issues proactively and optimize performance.
What Problems Can Data Discovery Solve?
- Identifying Market Trends: By analyzing customer behavior, market dynamics, and competitor activities, organizations can identify emerging trends and capitalize on market opportunities.
- Improving Operational Efficiency: Data discovery enables organizations to streamline processes, optimize resource allocation, and identify bottlenecks or inefficiencies in operations.
- Enhancing Customer Experience: By gaining insights into customer preferences, feedback, and sentiment, organizations can personalize products, services, and marketing efforts to better meet customer needs and expectations.
- Mitigating Risks: Data discovery tools can identify potential risks, fraud patterns, or compliance issues within data sets, allowing organizations to take proactive measures to mitigate risks and ensure regulatory compliance.
- Enhancing Decision-Making: Data discovery empowers decision-makers with timely and accurate insights, enabling them to make informed decisions based on data-driven evidence rather than intuition or guesswork.
- Optimizing Resource Allocation: By analyzing data related to resource usage, such as human capital, finances, and equipment, organizations can optimize resource allocation strategies to maximize efficiency and minimize waste.
- Identifying Opportunities for Innovation: Data discovery can uncover hidden patterns or correlations within datasets that may indicate opportunities for innovation or new product development, helping organizations stay ahead of the competition.
What Should You Look for in Data Discovery Tools?
- Scalability: Ensure that the tool can handle large volumes of data and scale to meet growing business needs.
- Ease of Use: Look for intuitive interfaces and user-friendly features that empower users across various skill levels to explore and analyze data effectively.
- Integration Capabilities: Choose tools that seamlessly integrate with existing data sources, systems, and BI platforms to maximize interoperability and data accessibility.
- Advanced Analytics Capabilities: Seek tools that offer advanced analytics, machine learning, and predictive modeling capabilities to uncover deeper insights and drive informed decision-making.
Data Discovery Steps
- Data Collection: This initial step involves gathering data from various sources, including databases, files, APIs, and external sources like social media or third-party vendors. It's crucial to ensure the quality, consistency, and completeness of the collected data, verifying its accuracy and relevance to the analysis objectives.
- Data Preparation: Once the data is collected, it needs to be prepared for analysis. This involves cleaning the data to remove errors, inconsistencies, or missing values, as well as transforming and integrating it into a unified format. Data preparation also includes standardizing data formats, resolving data conflicts, and handling outliers to create a clean and structured dataset suitable for analysis.
- Exploratory Analysis: In this phase, analysts explore the prepared data to gain an initial understanding of its characteristics and uncover patterns, trends, and relationships. Exploratory analysis involves using descriptive statistics, data visualization techniques like charts and graphs, and interactive tools to visually explore the data and identify interesting insights or anomalies that may warrant further investigation.
- Hypothesis Testing: Based on the insights gained from exploratory analysis, analysts formulate hypotheses or research questions to test using statistical methods or predictive modeling techniques. This step involves applying appropriate statistical tests, regression analysis, or machine learning algorithms to validate or refute hypotheses and uncover meaningful relationships between variables.
- Insights Generation: Once hypotheses are tested, analysts interpret the results to derive actionable insights, recommendations, or conclusions that address the original research questions or business objectives. This involves synthesizing the findings from data analysis into meaningful insights that inform decision-making and drive business outcomes, such as identifying market opportunities, optimizing operations, or improving customer experiences.
- Communication and Visualization: Finally, the insights generated from data analysis need to be effectively communicated to stakeholders. This involves creating interactive dashboards, reports, or presentations that present key findings in a clear, concise, and visually appealing manner. Data visualization techniques like charts, graphs, maps, and infographics are used to convey complex information intuitively, enabling stakeholders to understand and act upon the insights effectively.
In conclusion, data discovery is a fundamental process in business intelligence and analytics, enabling organizations to unlock the value of their data by uncovering insights and driving informed decision-making. By following the structured steps of data collection, preparation, exploratory analysis, hypothesis testing, insights generation, and communication, businesses can extract actionable insights from their data to gain a competitive edge, optimize operations, and enhance customer experiences.
At EnqDB we recognize the importance of data discovery in empowering organizations to harness the full potential of their data. Our platform provides advanced tools and capabilities for seamless data visualization, enabling businesses to uncover valuable insights and drive meaningful outcomes. With EnqDB, organizations can accelerate their data discovery process, streamline decision-making, and achieve their business objectives more effectively.